Last week I attended the Semiotic Machines conference here in Berlin. At the opening panel on the first day, the philosopher Beatrice Fazi said something that intrigued me and has kept me thinking ever since. She said – I’m paraphrasing from memory here – that in order to address the challenges that AI systems pose to our societies, the humanities need to do more thinking rather than more making.
I honestly didn’t understand what she meant. I don’t think that she was necessarily referring to, say, the Kantian reason when she defended thinking over making. The day after, I thought that maybe she was referring to the kind of critical thinking embodied in papers like the influential “On the Dangers of Stochastic Parrots” (Bender et al 2021). But recalling her initial intervention in that roundtable, and then reflecting on her response to Melanie Mitchell’s keynote on the second day, I concluded that neither was the case.
This opposition between thinking and making arose in the context of Fazi’s sideways reference to Kittler, who, according to her, studied and discussed media technologies by privileging making – an approach she called poietic. In other words, one is forced to understand that she was implying that the methods developed by Kittler are at least not sufficient to address the challenges posed by contemporary AI systems.
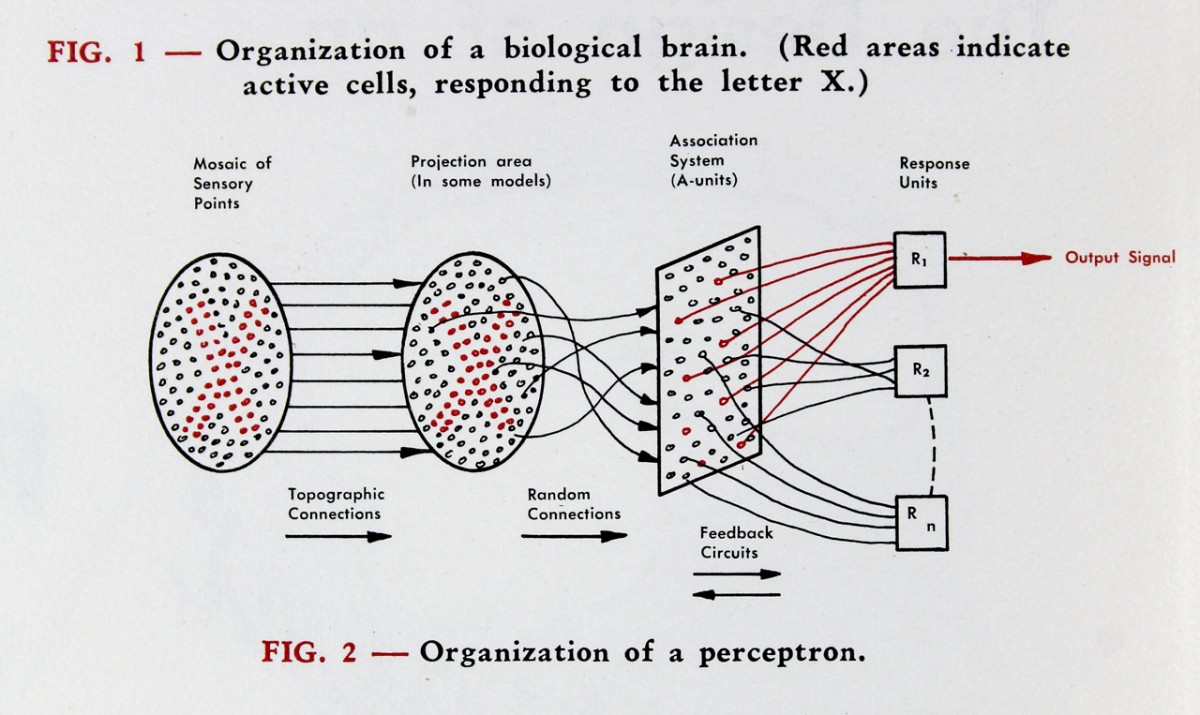
Of course, I could not resist the temptation and I asked her about it on the very first day of the conference. In retrospect, however, I see that my question was poorly phrased. I asked her whether, given the insights that we are now gaining from the development of AI models – for example, the big problems that self-driving vehicles are presenting to AI scientists, and from which Matteo Pasquinelli (2023) argues that there seems to be much more complex thinking and analysis than previously thought in social tasks such as truck driving, which were considered unskilled labor before these AI systems – it is still possible to draw a hard line between thinking and making.
Fazi replied that she meant more thinking in the humanities, not in AI development circles. Obviously, my question and skepticism was precisely about making this hard distinction between thinking and making in and for the humanities. I tried to explain myself, but I failed. So, a week later, maybe this note can help (me) a little.
***
I think it would be fair to say that in the context of German media studies – certainly located in a hybrid corner of the humanities – there is a general consensus about the relevance of making when it comes to investigating media technologies. In a way, this seems to refer to some foundational experimental endeavors, such as, of course, those developed by Friedrich Kittler – i.e., his construction of an analog synthesizer in the early 1980s (Döring and Sonntag 2020), as well as his programming experiments in computer graphics in the early 1990s (Holl 2017). But it’s certainly also part of a contemporary approach to the study of media, as it can be seen in the works of Christina Dörfling (2022) and Stefan Höltgen (2022), and more broadly, in other strands of media studies either in Asia, Europe, North and South America, in the works of Thomas Fischer (2023), Winnie Soon (2023), Mark C. Marino (2020), or Andrés Burbano (2023), to name a few.
However, the evident vastness of the AI question makes it impossible to confine this object of research to media studies alone, which is great, and both literary studies (see e.g., Weatherby 2022) and philosophy (see e.g., Hui 2021) are making it clear that they are on board – not to mention what is happening in the social sciences where the scholarly production on this topic is enormous (see e.g., the multinational research project Shaping AI).
But here I’m interested in sketching out a way to better understand Beatrice Fazi’s philosophical “dictum” in this regard; namely, that the humanities require more thinking rather than more making in order to grasp the scope of contemporary AI systems.
In her excellent 2020 paper, “Beyond Human: Deep Learning, Explainability and Representation,” Fazi argues that a key feature of AI systems – particularly those based on deep learning algorithms – is that they are radically removed from human thinking. This feature would not only obey their current inaccessibility – because the operations of these algorithms remain hidden in their deep layers – but, more fundamentally, it would respond to the fact that the modes by which these AI systems “abstract” and “represent” (for themselves) the inputs they are fed – which they pass from layer to layer in their internal processes – construct “new, complex worlds in equally new, complex computational ways” (Fazi 2020, 64) – worlds for which we would lack an adequate system of representation (see Fazi 2020, 59). In other words, a new epistemological regime would be being developed within the deep layers of these AI systems, and the distance between this new regime and ours would therefore constitute an equally new and challenging “incommensurable dimension” (Fazi 2020, 66).
Here I have to emphasize the clarity and simplicity with which Fazi explains the operations of deep learning systems in her paper – a clarity and simplicity that would allow even a non-initiated reader to understand how a deep learning AI system operates. This is relevant because in media studies, at least in its Berlin-based strand, the relevance ascribed to making lies precisely in the promise that through experimental making one would gain the necessary insights to really understand – and thus to explain – the properties of technological systems well. In other words, this promise would conceal the thesis that pure analytical reason is not sufficient to understand the complexities of contemporary technologies.
To accept this thesis would imply that Fazi must have used some sort of hands-on procedure to gain the necessary insights that allowed her to properly picture the operations of these systems. This is unlikely, however, because Fazi concludes her paper with a cautionary note: opening the black box of AI – as the Berlin School of Media Theory openly invites us to do – can lead to the risk of finding it empty, or more simply, of finding “nothing to translate or to render precisely because the possibility of human representation never existed in the first place.” (Fazi 2020, 71). In other words, according to Fazi, before opening the black box of AI, we should develop a “theory of knowledge specific to computational artificial agents.” (Fazi 2020, 69).
But how could we develop such a theory of knowledge without having a clear picture of how these agents operate? The problem seems gigantic to me, and in a way brings me back to the same point I was at when I heard Fazi say that the humanities need more thinking rather than more making in order to fully grasp the scope and scale of AI systems.
***
In what may at first seem a little bit off-topic, I spent last weekend reading some essays by the late Marina Vishmidt – my tri-weekly readings for the counter-infrastructures reading group at diffrakt, in which we discuss the extent and scope of infrastructures and their opposite(s). Vishmidt’s essays, which are part of a larger discussion about the role of capital and labor in the art world, propose a shift from “institutional critique” to “infrastructural critique.” As I read these texts, two things caught my attention and led me to return to my ruminations on the question of thinking and making prompted by Fazi’s words.
First, in this invitation to move from institutional critique – e.g., the museum, the university, etc. – to infrastructural critique, Vishmidt defines infrastructure as “a conceptual diagram that enables thought to develop” (Vishmidt 2017, 265). To develop what (kind of thought), one might ask? As it’s possible to determine after reading a couple of other pieces (Vishmidt and Petrossiants 2020; Vishmidt 2021), to develop a critique of the material conditions of power and labor in institutions like the museum.
The second aspect that caught my attention was the thick Hegelian and Adornian metalanguage that completely crams Vishmidt’s proposal. For non-Hegelians (and I’m certainly not one), it might seem that Vishmidt’s invitation consists of three layers: 1) at the top, a hardcore theoretical framework that establishes what thought, abstraction, identity, negativity, and so on are – the Hegelian layer, one might say; 2) the infrastructure as a diagrammatic plan that leads to visualizing how the institution operates; and 3) the institution itself. Moreover, it seems to me, the proposed shift works on a top-down dynamic, that is, it is the upper theoretical framework that is coupled, via the middle layer, to the institution in order to think critically about it – to analyze it.
But what does all this have to do with the question of thinking versus making, and with Fazi’s approach to contemporary AI systems? I think that Vishmidt’s infrastructural critique can help us see that what is at stake here, in the challenge of how to analyze and think about contemporary AI systems, is the question of the direction in which the analysis falls. In a word, a question of deduction versus induction.
What I mean is that Fazi’s warning that the humanities, before opening the black box of AI machines, must develop an ad hoc theory of knowledge in order to properly analyze whatever we find there, might imply that we inadvertently fall prey – as Vishmidt apparently did via Hegel and Adorno – to the persistent conviction that it is only within human reason and its old spirit that such a theory can originate.
On the opposite side, German media studies seem to have proposed a hardcore inductive approach. That is, let’s open the black box a let the theories we might need emerge from the machines themselves. I have the impression that Fazi had this position in mind when she said that the humanities need more thinking rather than more making. After all, (we) Kittlerians believe that everything that can supposedly originate in human thought, is just the unconscious, “imaginary interior view of media standards” (Kittler [1993] 1997, 132).
But ironies aside, I really think that we face a complex conundrum here. If we develop a thorough (human) theory of knowledge for understanding the processes of abstraction and conceptualization deploy by AI systems, one that although radically different still draws on the centuries-old tradition of reason and abstraction bequeathed to us by the humanities, we certainly risk inadvertently disguising aspects of ourselves as machinic. On the other hand, if we allow this new theory to emerge from the machines themselves, we may well confuse the emergence of pure noise with this so expected and needed theory.
Without a thoroughly proven solution yet, I think it’s precisely Marina Vishmidt’s work that can help us overcome this conundrum – and thus also overcome the problematic divide between thinking and making. I’m referring to Vishmidt’s second layer, as I put it above, that is, infrastructure as a diagrammatic plan. If we open the black boxes of deep learning systems, and later of the technologies to come, and draw diagrams of their architectures and modes of operation – I wonder if Beatrice Fazi studied diagrams of these systems to build her clear understanding of them – we can outline the way to draw new diagrams that connect these operations to the old frameworks that drive our reasoning. In other words, the development of a language of abstractions and operations in the middle zone between our existence and that of machines.
After all, diagrams are strange creatures – half the product of thinking, and half the product of making.